Companies to Watch Using AI in Drug Discovery
Search For Schools
When you click on a sponsoring school or program advertised on our site, or fill out a form to request information from a sponsoring school, we may earn a commission. View our advertising disclosure for more details.
“Companies that learn how to convert theoretical and computational predictions into high-quality action following standard regulatory data validation will succeed because they will rapidly develop new products. I am less optimistic about companies solely focusing on their own AI and not transitioning from a tool to the practical utility of that tool.”
Alexander Tropsha, PhD, K.H. Lee Distinguished Professor, Eshelman School of Pharmacy at the University of North Carolina at Chapel Hill
Historically, drug discovery has been characterized by high costs, lengthy timelines, and considerable uncertainty. Traditional methodologies often rely on trial and error, involving extensive laboratory research, animal testing, and multiple phases of human clinical trials. This painstaking process can span over a decade and requires significant financial investment. Yet, the success rate for drugs to make it from discovery to market remains low, with only 10 to 15 percent of drugs actually making it to market.
However, artificial intelligence (AI) is poised to change that. With AI, pharmaceutical companies can accelerate the drug discovery process, enhance the precision of targeting specific diseases, and significantly reduce the time and cost associated with bringing new medications to the market. AI’s ability to analyze vast datasets and uncover patterns invisible to the human eye opens up new opportunities for personalized medicine and novel treatments.
“If you look at the traditional drug discovery pipeline, the biological experiments increase in complexity as we move through higher levels of species up to animals, and then finally, we are allowed to test them on humans,” explains Dr. Alexander Tropsha, K.H. Lee distinguished professor at the UNC Eshelman School of Pharmacy at the University of North Carolina at Chapel Hill. “I think to the point of just having new chemicals and forecasting the probability of getting to a clinical trial, that’s where AI can be helpful. Also, it can help with broad global analysis of all the relevant information and summarization.”
Large language models (LLMs), AI trained with a vast set of data, and statistical modeling, which is what AI is built on, are both areas where AI is proving useful. “LLMs are used to formulate the direction in which chemists should pursue their studies. At the right time, you can tune quantitative and statistical models to answer specific questions that are beyond linguistic capabilities,” says Dr. Tropsha. One such example of this is ChemCrow, which does exactly that—combines an LLM and statistical modeling for more accurate chemical discovery.
Those who can figure out how to leverage AI for drug discovery will have an advantage over the competition. “Companies that learn how to convert theoretical and computational predictions into high-quality action following standard regulatory data validation will succeed because they will rapidly develop new products,” says Dr. Tropsha. “I am less optimistic about companies solely focusing on their own AI and not transitioning from a tool to the practical utility of that tool.”
As with all AI, there are some concerns: “Trust but verify. Science has become closer in the minds of the general public. They understand the process and the results. However, they often get excited about it, leading to a lot of general interest. These tools appear easy to use, which can result in very misleading results. We should move forward with respect and deep understanding,” encourages Dr. Tropsha.
Keep reading to learn about AI drug discovery, recent developments, and ethical concerns.
Meet the Expert: Alexander Tropsha, PhD
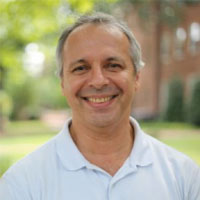
Dr. Alexander Tropsha is the K.H. Lee distinguished professor at the Eshelman School of Pharmacy at the University of North Carolina at Chapel Hill. With expertise in computational chemistry, cheminformatics, and structural bioinformatics, Dr. Tropsha’s research focuses on computational drug discovery, cheminformatics, computational toxicology, and health informatics.
Dr. Tropsha’s contributions to biomolecular informatics and dedication to understanding relationships between molecular structures have made him a respected figure in the academic and scientific communities. He is a prolific author with over 190 peer-reviewed papers and 20 books and chapters.
What Defines AI For Drug Discovery
At its core, AI for drug discovery involves using artificial intelligence and machine learning techniques to analyze vast amounts of data to uncover patterns and make predictions about potential drugs. This includes using large language models (LLMs) and statistical modeling to identify and develop new chemical compounds and utilizing AI algorithms to optimize clinical trial design and identify promising treatment targets.
Currently, AI is a buzzword that can often be misunderstood. “People call it AI, but in reality, it’s something that they’ve been doing for many years. There is a lot of misuse of the term AI as applied to drug discovery,” explains Dr. Tropsha. More traditionally, machine learning has been used in association with AI but there is a key difference, which is that an AI algorithm incorporates procedures that enable it to make independent decisions.”
He continues, “The thing is, machine learning-based models did not have this ability to process data in an ongoing way. You take the existing data, build a model, optimize the model, and then you have a fixed model. And so there’s no dynamic process. That, to me, is a discriminating factor. AI, on the other hand, can draw conclusions as it processes and validate those decisions for accuracy at the same time.”
The Future of AI in Drug Discovery
As technology advances and we gain a greater understanding of how to apply AI techniques to drug discovery, the potential for groundbreaking discoveries and treatments becomes increasingly more likely. “It will become very specific, and that gets less and less exploratory, more and more pragmatic,” says Dr. Tropsha.
One area that shows a lot of promise is generative chemistry, a revolutionary approach within the drug discovery process, harnessing the power of artificial intelligence to generate novel chemical structures with potential therapeutic properties. “Generative chemistry will continue to develop more and more structures in conjunction with a better understanding of chemical design rules and chemical synthetic groups. Bigger datasets will contribute to generating not just new chemical structures but new chemical structures that are increasingly realistic,” explains Dr. Tropsha.
“The biggest change has been in chemical companies that traditionally will synthesize chemical libraries that pharma companies would buy and test in the hopes of finding chemicals to develop into a commercial drug. Those libraries have grown in size dramatically in a very short period of time. We have gone from less than a billion molecules to now close to 50 billion molecules in just one year. In contrast, it took 20 years to reach the initial 1 billion molecules that can be made, enumerated, and used for computational drug discovery.”
“My expectation is that generative models will hallucinate about chemicals that can be made and then test to see if they can be made using structural rules, but not synthetic rules. This means they can be really hard to make,” he adds. “I expect that as these AI models learn enough rules of organic chemistry through the use of large language models, it will allow us to use them to produce more and more realistic and measurable chemical molecules. And not only make them but ensure they have desired properties at the time of synthesis. That’s the holy grail of the pharma industry.”
This process can drastically speed up drug discovery in many ways. “The primary way is that it will reduce the amount of experimental effort needed. AI can narrow results down to a smaller number of chemicals to validate theoretical predictions,” Dr. Tropsha explains. “It’s a shift from exploratory methodologies and drug discovery to more rational design.”
Recent Developments In AI That Will Affect Drug Discovery
Recent developments in artificial intelligence (AI) have significantly impacted the field of drug discovery, particularly in the realm of protein. “The biggest development has been the creation of AlphaFold from Google DeepMind. It didn’t solve the long-standing problem of figuring out how proteins rapidly fold from a primary sequence into a unique three-dimensional structure, but rather improved dramatically the accuracy of prediction of protein structures,” shares Dr. Tropsha.
“People are still exploring whether it’s practical for drug discovery. This theoretical method predicts the three-dimensional structure of the protein and enables the use of special computational tools. The current assessment is that the accuracy of this prediction—while the overarching shape prediction is accurate—is not precise enough at the clinic resolution level to affect drug discovery application. I think, in the next few years, the accuracy of the predictions will increase and will lead to more targeted development of new molecules with less effort faster and will get the compounds that have the desired activity.”
The ramifications of these advancements are profound. “Currently, there it’s still significant experimental effort required to characterize the three dimensional structure of a protein. As methods improve, instead of wading through the fairly substantial experimental effort of trial and error, we could instead rely on theoretical models of the project that will be accurate. That would be a really dramatic breakthrough,” he says.
Other developments include more familiar AI programs like ChatGPT. “Large pharma companies are increasingly using ChatGPT to integrate large scale and different levels of complexity data, to be able to extrapolate what happens in vitro to what we observe in vivo,” explains Dr. Tropha. “The number of molecules that are active in in vitro biological experiments is enormous. However, there is still a lack of understanding on how the initial experimental data in support of the drug discovery pipeline effectively translates through the entire drug discovery pipeline. With AI we can have a better understanding of this and extrapolate better.”
Top Companies Using AI for Drug Discovery
Here are some top companies currently working in the AI drug discovery space:
- Atomwise – Atomwise utilizes artificial intelligence to pioneer the use of deep learning algorithms for the structure-based discovery of new drugs. Their AI-driven platform, AtomNet, specializes in predicting the binding of small molecules to proteins, which is crucial for identifying novel drug candidates faster and with higher precision.
- BenevolentAI – BenevolentAI stands out for integrating artificial intelligence with drug discovery and development processes. Their unique AI platform leverages machine learning to understand the complexities of disease biology, identify potential drug targets, and accelerate the path from dynamic research insights to clinical development.
- DeepMind – A subsidiary of Alphabet Inc., DeepMind excels in leveraging AI for multiple complex challenges, including drug discovery. Their breakthrough in protein folding, demonstrated by AlphaFold, represents a significant leap forward in understanding protein structures, an essential component of developing new and effective drugs.
- Insilico Medicine – Insilico Medicine is at the forefront of using AI for drug discovery and development, especially focusing on age-related diseases. They employ deep learning techniques for drug target identification, generative chemistry, and predictive analytics to design molecules from scratch, significantly reducing the time needed for early-stage drug discovery.
- Recursion Pharmaceuticals – Recursion Pharmaceuticals combines artificial intelligence with experimental biology to decode biology and drive drug discovery. Their proprietary platform uses automated, high-throughput screening of cellular phenotypes, applying machine learning models to predict the efficacy of compounds, streamline the drug discovery process, and identify treatments for rare diseases.